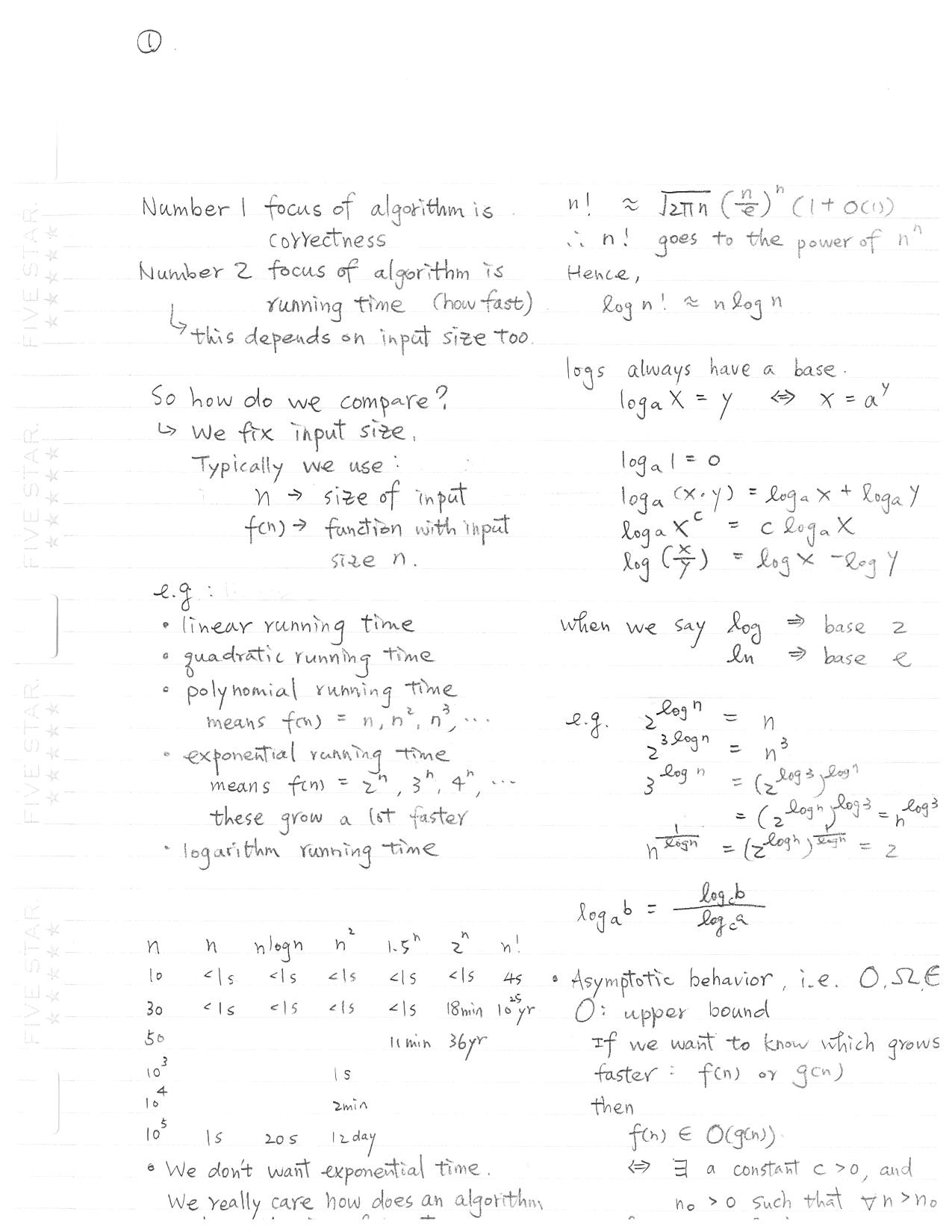
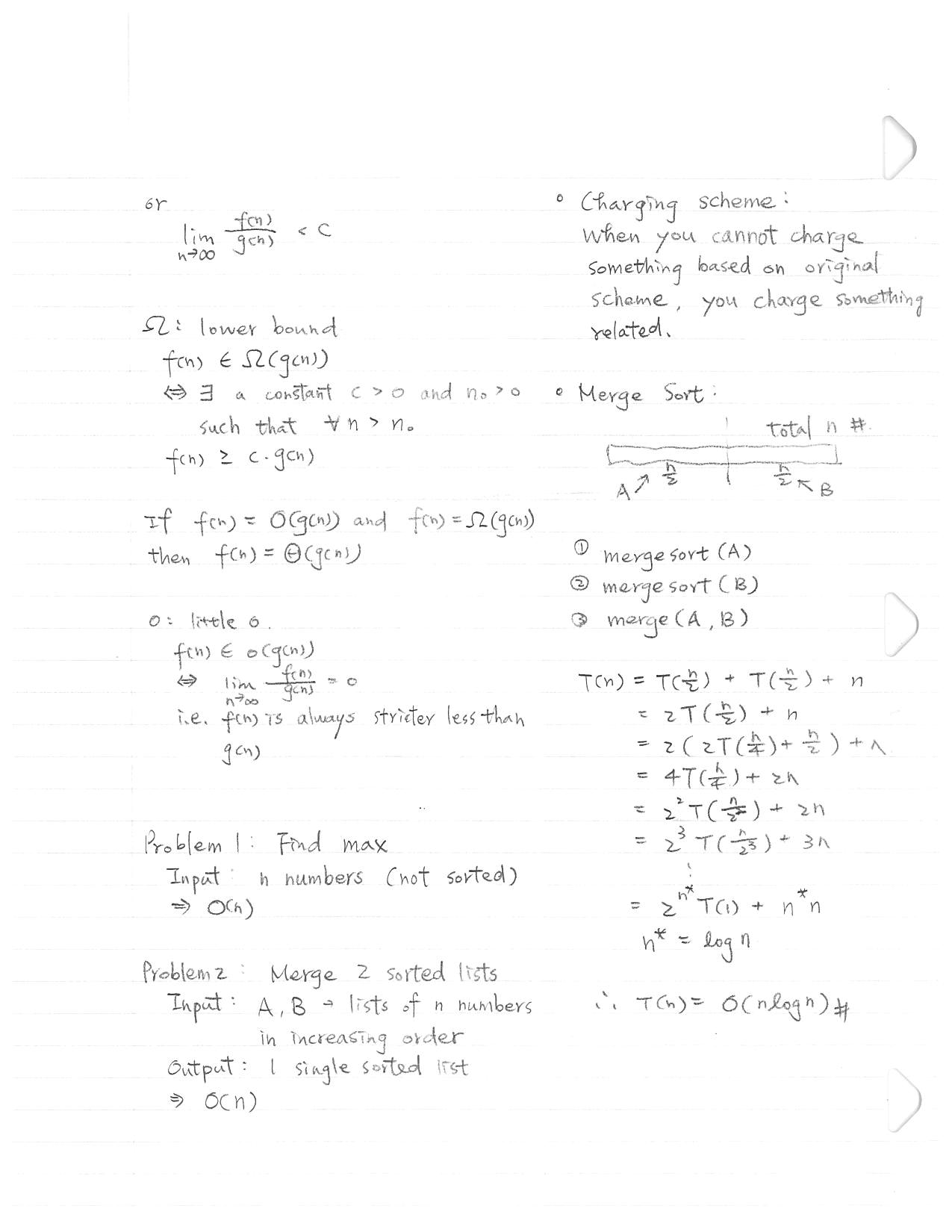
What should we focus on when we are designing an algorithm?
No. 1 focus of algorithm is correctness
No. 2 focus of algorithm is the running time (how fast can this algorithm be)
This depends on input size too.
So how do we compare?
We first fix input size and then
Typically we use n as the size of input
And use f(n) as the function with input size n
e.g.
Linear running time: f(n) = 2n
Quadratic running time: f(n) = n^2
Polynomial running time: f(n) = n, n^2, n^3, n^4, ….
Exponential running time: f(n) = 2^n, 3^n, e^n, …
Exponential running time grow a lot faster
Logarithm running time: f(n) = log n, ln n, log_3 n, log^2 n, log (log n), log^* n
(log^* n => the bnumber of logs taken before we get a value is less than 1)
Rule of thumb:
We don’t want exponential running time.
We really care how does an algorithm scale with size of input.
n! approximately equals to (2 Pi n)^(0.5)(n/e)^n (1 + O(1))
=> log (n!) approximately equals to n log n
Asymptotic behavior
- Upper bound: big O
- Lower bound: big Omega
Running time exercises
Find max
Input: n numbers (not sorted)
Running time: O(n)
Merge two sorted lists
- Input: A, B are two lists of n numbers in increasing order
- Output: one single sorted list
- Running time: O(2n)
Charging scheme
- When you cannot charge something based on original scheme, you charge something related
Merge sort (divide and conquer techniques)
Input: n numbers (not sorted)
Output: n numbers (sorted)
Running Time: O(n log n)
Strategy:
divide original list into two lists A and B
mergesort(A)
mergesort(B)
merge(A and B)
Closest pair problem
Problem: n points in the plane with (x, y) coordinates, find 2 points closest in distance
Solution: find all distance and find max of them
i.e. C(n, 2) => O(n^2)
Independent set problem
What are independent sets? They are subset of points which do not conflict. i.e. set of vertices that have edges in between.
Problem: Check if the given graph G has k independent vertices.
Solution:
- Find all possible subsets with k vertices
- Check if each set satisifies
No. of subsets = C(n, k) = n!/()(n - k)! k!) => O(n^k)
Maximum independent set problem
- Problem: Give the largest independent set of k vertices.
- Solution: use previous algorithm and try for all k. i.e. C(n, 1) + C(n, 2) + … + C(n, n) = 2^n (exponential running time)
Traveling salesman problem
- Problem: A sales man travels to n cities. The distances between the cities are given. Find shortest tour of n cities.
- Solution: Enumerate all possible paths, i.e. n! ~ n^n
Binary search (Sublinear algorithm, grows slower than linear)
- Problem: Is a number q in the sorted n numbers?
- Solution: Each comparison throws away half the numbers. => O(log n)